Forecasting Trends: Using Predictive Analytics in Your Data Analysis App
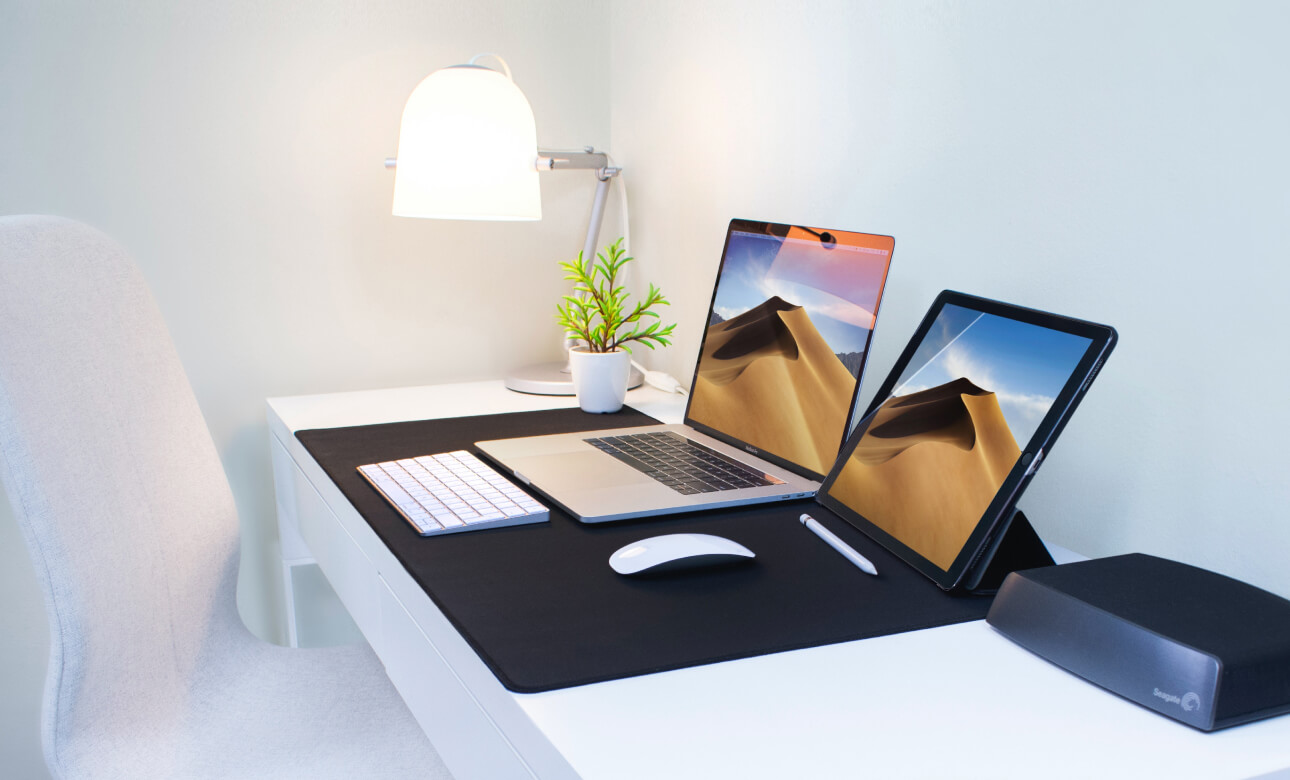
In today's data-driven world, businesses and individuals alike are constantly seeking ways to gain valuable insights from their data. Predictive analytics has emerged as a powerful tool for anticipating future trends and making informed decisions. In this blog post, we will explore the significance of predictive analytics and how it can be integrated into your data analysis application to forecast trends accurately.
Understanding Predictive Analytics:
Predictive analytics involves extracting information from historical data to identify patterns, trends, and relationships that can be used to make predictions about future events. By applying statistical algorithms and machine learning techniques to past data, predictive analytics models can provide valuable insights into what might happen next. These insights are invaluable for decision-making, risk assessment, and strategic planning.
The Importance of Forecasting Trends:
Forecasting trends is crucial for businesses to stay ahead of the curve. Whether it's predicting customer behavior, demand for products, financial market trends, or even weather patterns, accurate predictions provide a competitive advantage. By understanding upcoming trends, businesses can allocate resources effectively, adjust their strategies, and make proactive decisions rather than reactive ones.
Integrating Predictive Analytics into Your Data Analysis App:
- Data Collection and Cleaning:The foundation of any predictive analytics model is high-quality data. Ensure that your data analysis app collects relevant and accurate data. Additionally, invest in data cleaning and preprocessing to remove inconsistencies and outliers that could affect the model's accuracy.
- Feature Selection:Identify the most relevant features (variables) that are likely to impact the outcome you're trying to predict. Applying domain knowledge and statistical techniques can help you select the right set of features for your predictive model.
- Choosing the Right Model:Depending on the nature of your data and the problem you're solving, you'll need to choose an appropriate predictive model. Options include linear regression, decision trees, neural networks, and more advanced techniques like ensemble methods or deep learning.
- Training and Validation:Split your data into training and validation sets to train and test your predictive model's performance. This step helps you identify if your model is overfitting (performing well on training data but poorly on new data) or underfitting (not capturing the underlying patterns).
- Feature Engineering:Enhance your model's performance by creating new features derived from existing ones. Feature engineering can uncover hidden patterns and relationships in the data, leading to improved predictions.
- Regular Updates:Trends can change over time, and the predictive model's accuracy can degrade as new data becomes available. Implement a system to periodically update and retrain your model to ensure it remains relevant and accurate.
- User-Friendly Interface:Your data analysis app should present predictions and insights in an easy-to-understand format. Visualizations, graphs, and intuitive explanations can help users comprehend and utilize the predictions effectively.
Benefits:
- Informed Decision-Making: Predictive analytics empowers users to make informed decisions based on future predictions.
- Competitive Edge: Anticipating trends gives businesses a competitive advantage by enabling proactive strategies.
- Resource Allocation: Accurate forecasts help allocate resources efficiently, reducing waste and costs.
- Customer Insights: Predictive models can reveal insights into customer preferences and behaviors.
Challenges:
- Data Quality: The accuracy of predictions heavily relies on the quality of input data.
- Model Complexity: Developing accurate predictive models often requires a deep understanding of machine learning techniques.
- Continuous Learning: Predictive models require regular updates to adapt to changing trends.
Conclusion:
Integrating predictive analytics into your data analysis application opens up a world of possibilities for accurate trend forecasting. By harnessing the power of historical data and sophisticated algorithms, businesses and individuals can make smarter decisions, stay ahead of the competition, and navigate the future with confidence. Remember that successful predictive analytics requires a combination of domain knowledge, technical expertise, and a commitment to continuous improvement.